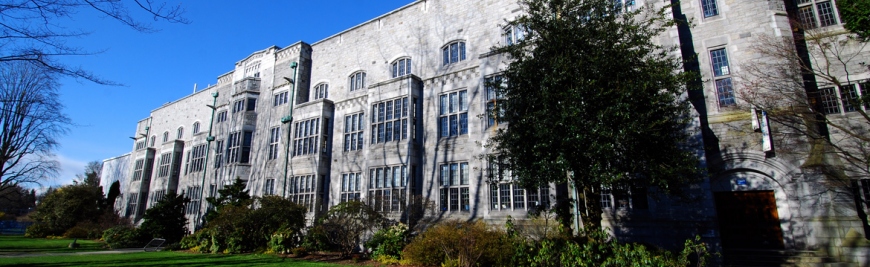
Jolene Reid
Profile
Featured Publications
Research and Teaching Interests
Our group uses both computation and experiment to understand and predict reaction outcomes in organic chemistry.
Data-driven Reaction Optimization
Chemical syntheses are generally devised by practitioners with significant experience and yet, are often executed in a trial-and-error fashion. In such scenarios, the practitioner will often evaluate a number of variables (catalyst, substrates, reagents, solvents, etc.) related to the target transformation, often one at a time, however, such a process is iterative and resource intensive. Importantly, it is not scientifically rewarding, as the primary reasons for reaction performance are not frequently revealed, hindering hypothesis-driven idea development and applications of a reaction to more complex substrates. Thus, the overarching objective of our program is to apply data-driven workflows incorporating physical organic principles directly within the reaction development and discovery process. Our approach to this is focused on three key aims:
(A) Machine learning applications to reaction development
(B) Discovery of new and efficient catalyst systems
(C) Automating reaction condition selection
Analyzing Reaction Mechanism
The development of effective catalytic processes relies on an understanding of the key interactions between catalysts and substrates at the relevant transition states. Consequently, the calculation of transition states involved in these processes and attempting to understand the interactions that give rise to performance have become integral steps in the workflow for reaction development. Therefore, one of our key aims is to identify the important noncovalent interactions that guide enantioselectivity outcomes in modern organic reactions. Based on fundamental mechanistic insights, novel applications and catalysts can be designed.